3 Cs of Data Quality
When you're figuring out how to measure your data quality, there's a lot of guidance out there.
A lot of it is framed in terms of dimensions of data quality. Dimensions are definitely a useful framing device for conceptualizing and aggregating data quality in important ways.
There's no set of data quality dimensions that is recognized as a universal standard. While this is OK, it can make it hard to get started.
Here's our suggestions of what are the core data quality dimensions to start with. We've divided them into three related categories: completeness, correctness, and clarity.
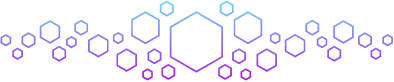
To envision how all these fit together, imagine that your data is pieces of a puzzle.
To get value out of your data, you need to assemble the puzzle (do data quality).
Completeness = having all the pieces to complete the puzzle shape.
Correctness = having all the pieces be from the same puzzle.
Clarity = having the image on each puzzle piece be intact.
Completeness
Key idea: Your data is describing something—people or places or things or some combination of those.
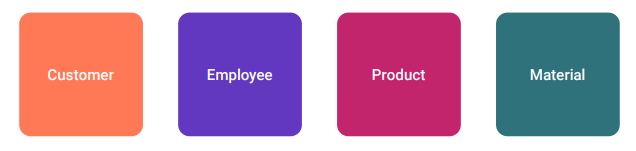
Completeness is about how your data describes those objects:
Existence
- Does it contain the data you need?
- Does it have the level of detail you need?
and
Accessibility
- Can you get to it?
Correctness
Key idea: The something your data is describing is a real-life something.
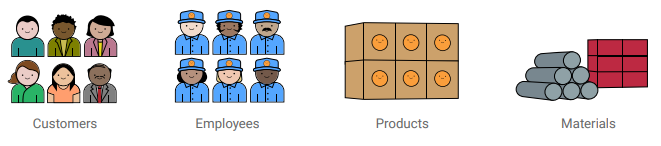
Correctness is about your data's fidelity to the real-life objects it is describing:
Accuracy
- Does the data about the entity reflect its real-world characteristics in a way that is suitable for your usage of the data?
and
Recency
- Is the data recent enough that you are confident in it?
Clarity
Key idea: The something your data is describing has more than one aspect, and it has connections to other objects, too—it's not just floating in a void.
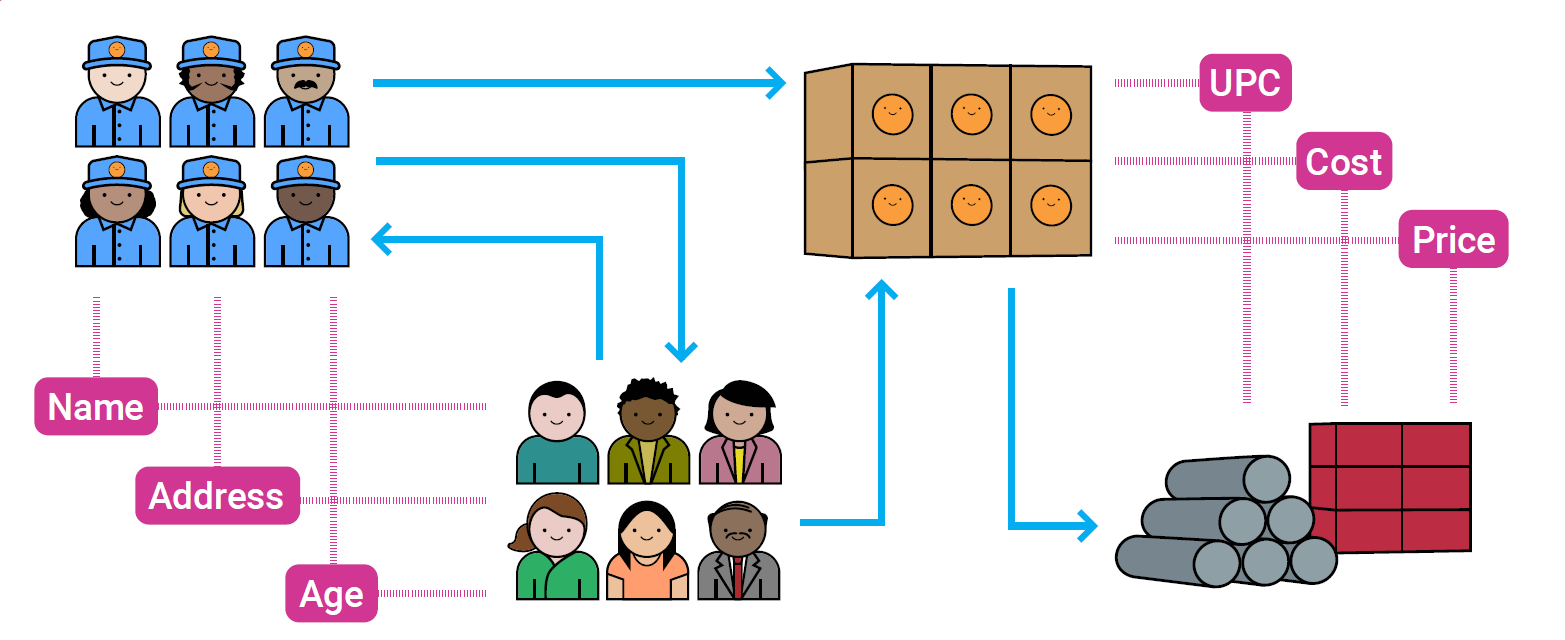
Clarity is about understanding the different aspects of the entity and how they relate to each other, as well as how the entity relates to other entities:
Readability
- Is the data comprehensible to the people and systems that need it?
and
Standardization
- Is all data that is similar comparable, no matter where it occurs?
and
Connectivity
- Do you have the data to recognize when there is data about a single entity in multiple places in a way that is relevant to you?
- Do you have the data to recognize when there are relationships between entities that are relevant to you?
If you feel like you've gotten everything you were looking for by this point, great! We're glad we could provide you with some direction.
But if you (or stakeholders you have to answer to) want a more specific breakdown, download the full ebook for more information about how we define the different dimensions.