Meet the trailblazer approach to data quality
If you’re trying to introduce data quality in a small or medium organization, this article is for you. Because it’ll tell you how to get started on data quality when you don’t have many resources.
(This’ll still work for you too, big-company people. Especially if bureaucracy is an obstacle for you.)
Where the big names break down
Pick up a standard data quality reference, or a top google search result, and you’re likely to see one if not both of these things:
- A series of suggested steps for applying data quality to all of your data. All of it. Right now.
- A recommendation that you form a DQ council, or hire dedicated data quality staff, or both.
If you’re at a small or medium company, or you have no significant budget at a company of any size, both of these are borderline unachievable in any reasonable timeframe. Unless you’re willing and able to turn all your operations upside-down to make it happen, which: why would anyone want that?
Especially when there’s a better way?
Why data quality is hard
If you don’t have the budget and/or authority to:
- Require people to add data quality work to their existing duties and prioritize it
- Hire dedicated data quality staff
The reason that it’s hard to achieve those things is obvious and we will not be discussing it further here.
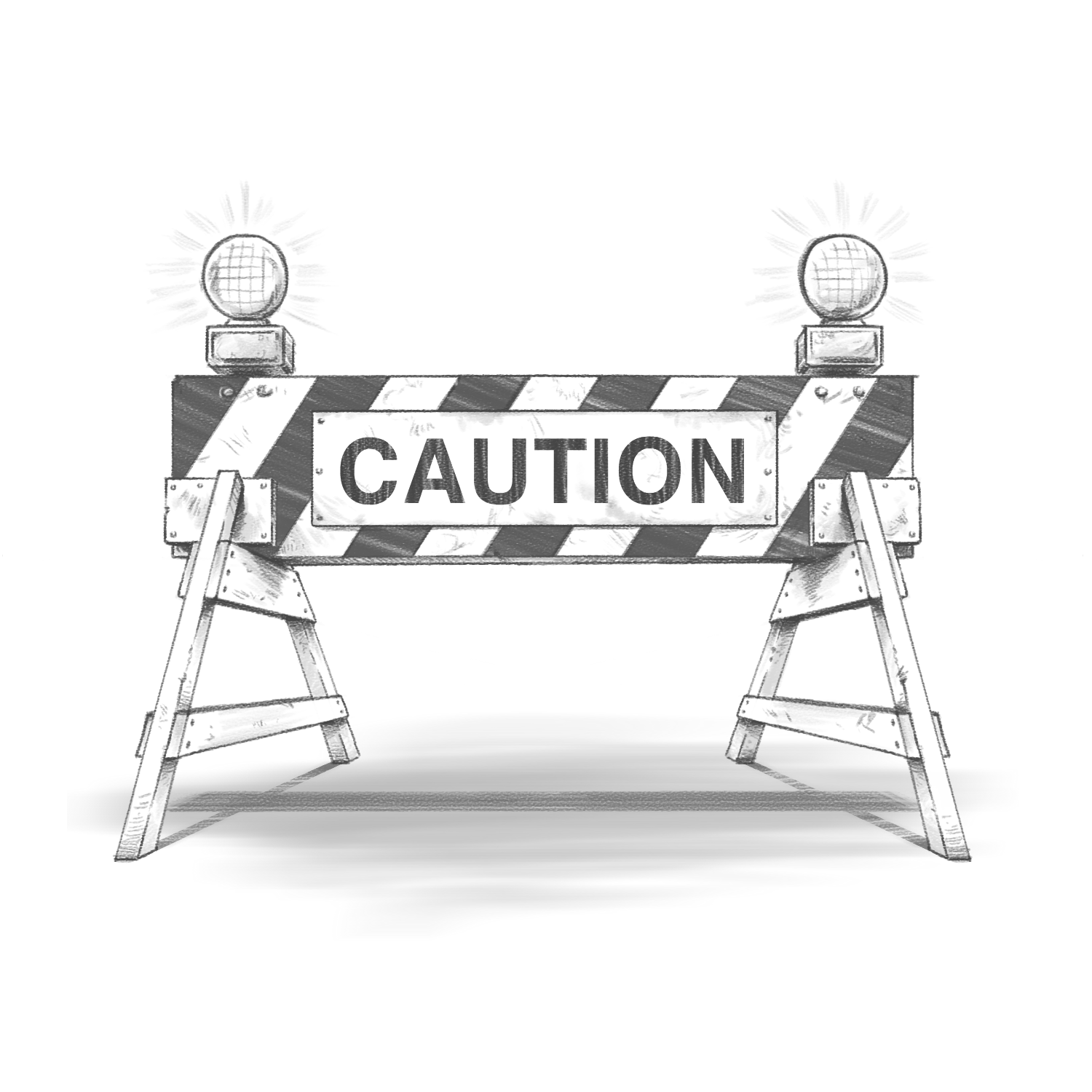
“Applying data quality to all your data” sounds a little more benign. Even advantageous: isn’t having a clear journey laid out for your project good? You know where you’re going.
The problem is that this top-down approach overlooks some major challenges.
What’s your goal?
You need to know your destination if you want to make a usable map. And the goal of data quality is often defined as something like “making the data fit for use.”
But use by who? Data scientists? Customer service representatives? The consultant that responds to GDPR queries? Your marketing intern?
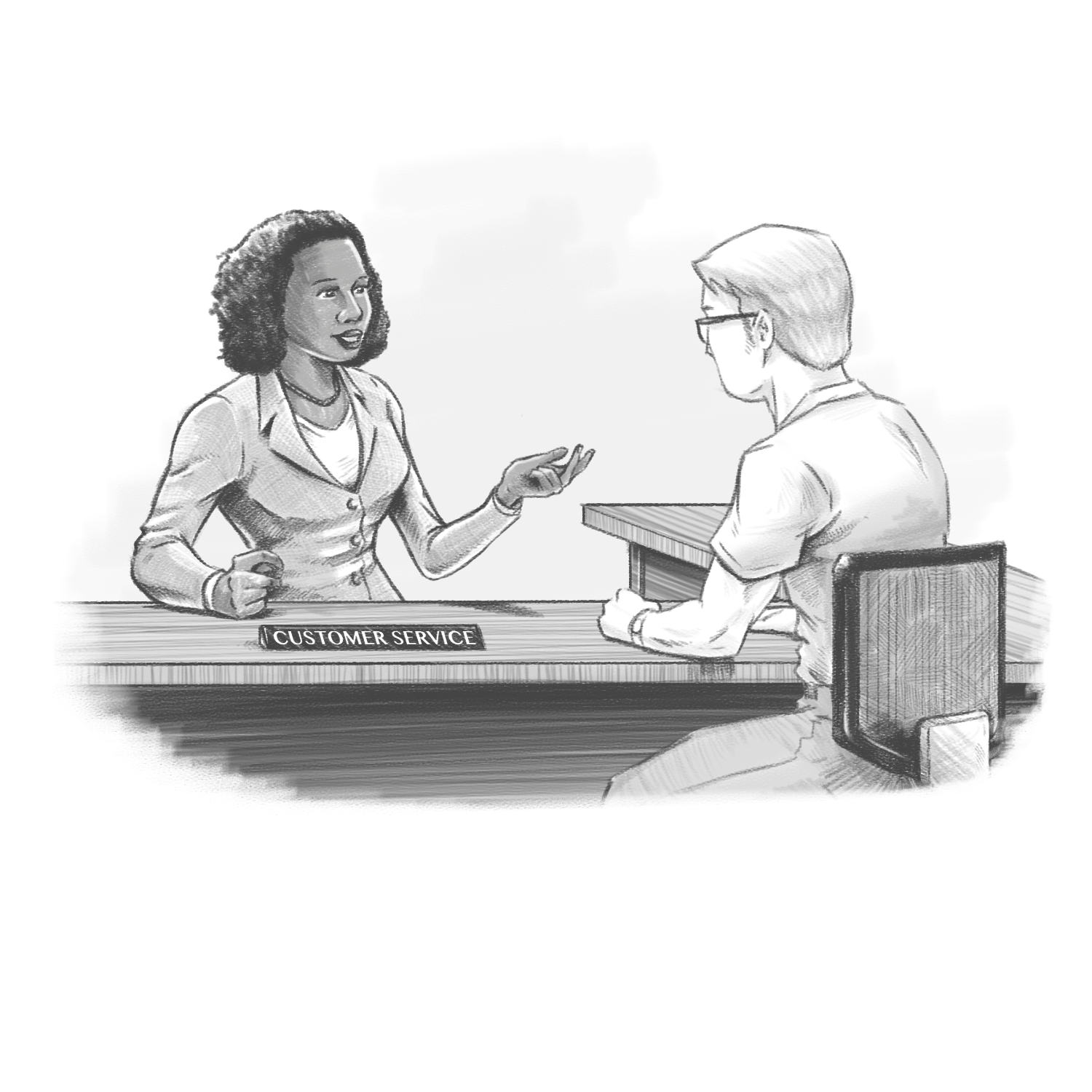
Even if you know all those answers off the top of your head, there are nuances about what fit for use means to each of those groups that vary depending on who is using the data and what they’re doing with it.
Is your address data just used for geographical stats or do you need it to be USPS-ready for mailing? Is city+state good enough or do you need streets and numbers?
How up-to-date does your data have to be? How long does it take users to get it? Is a one-week lag in acquisition time OK for their purposes? What about a day? An hour? A minute?
You need that level of detail, and even more, if you want to make a top-to-bottom picture of your DQ requirements.
What do you have?
It’s not uncommon for an organization to not know about all of the data it has available. Even at small companies, it’s all too easy for business practices to diverge from whatever the software was designed for just a little bit.
And that’s how a crucial piece of data ends up in a field called “Notes.”
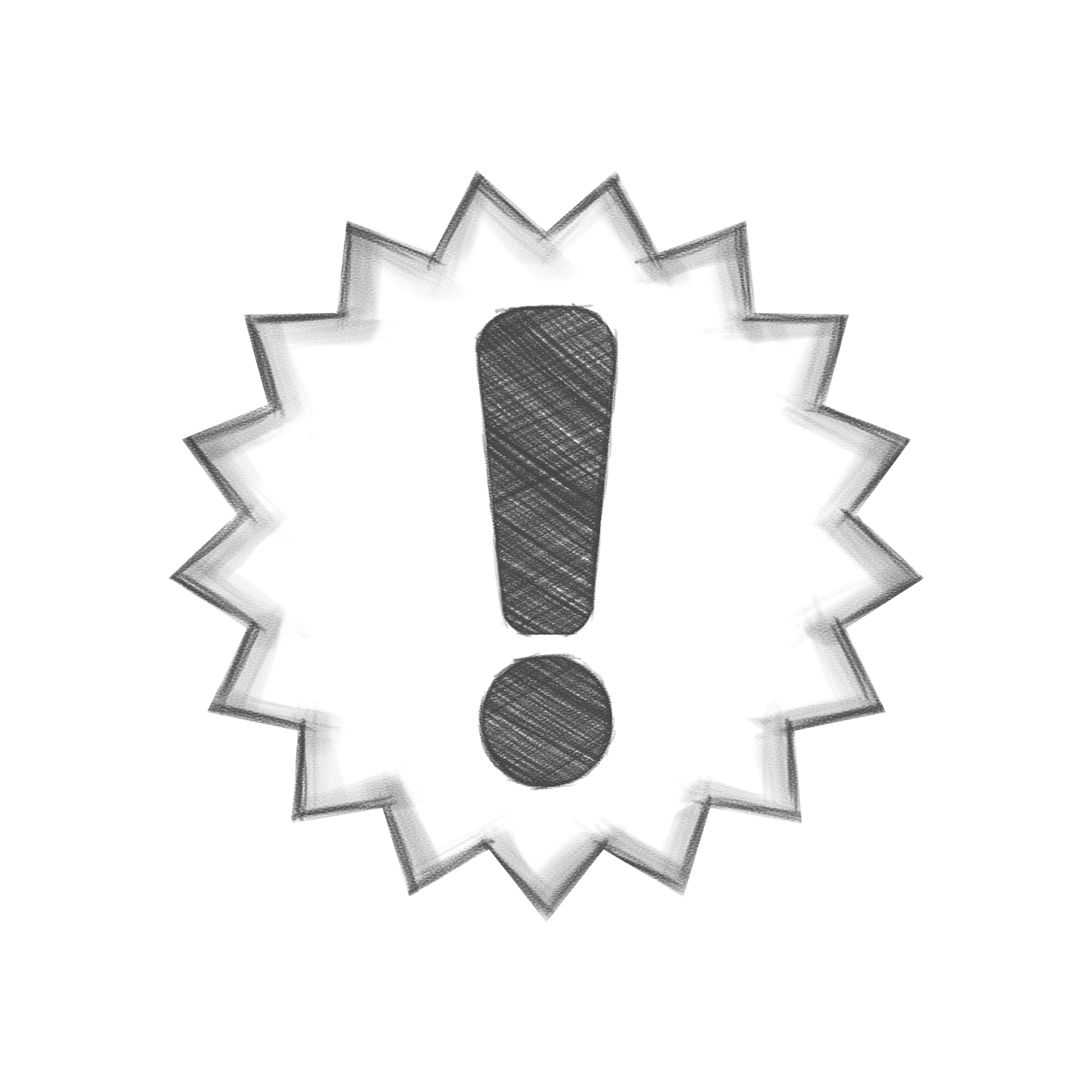
The company not knowing about the data doesn’t mean literally no one knows, of course. The people who work with the data and system on a day-to-day basis know exactly where it is.
But you have to talk to all those people before you can make the plan.
Plus you have to deal with data that you can’t get to. Maybe the system is so old and slow that by the time you can get the data out it’s not relevant anymore. Maybe the format is from ancient times and you can get the data out but not use it.
The amount of data you have can be a challenge too.. Even if your data volumes don’t meet the classic definition of big data, when you don’t have much data experience the definition of “big” gets small really fast.
How can you make this plan?
Now all of the big names are recommending that you make a top-down map of where your data quality project needs to go, and you don’t know exactly what data you have, what you need it to look like, if you can even get there.
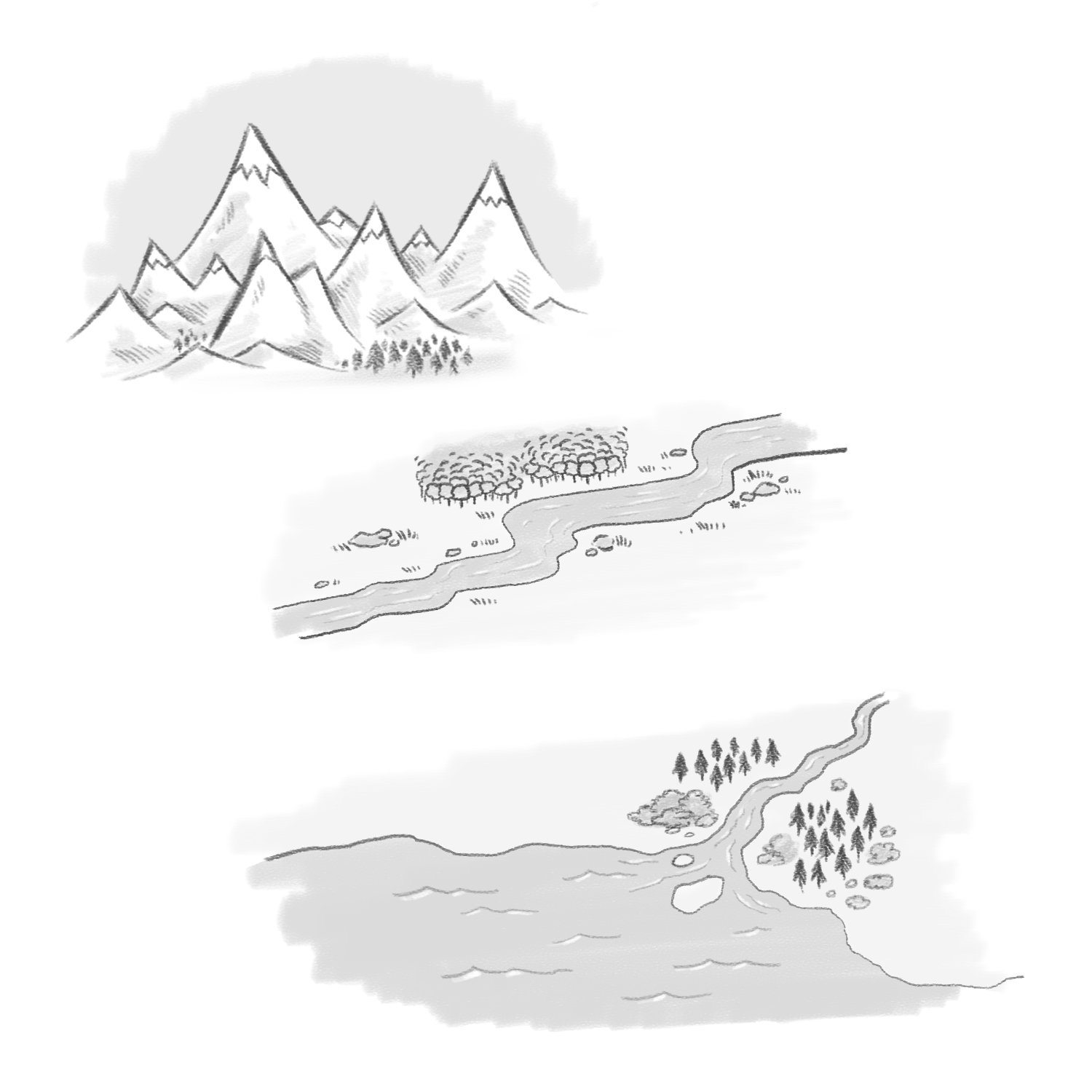
That introduces a lot of uncertainty. You need to expect challenges will arise from that uncertainty. And they won’t be one-at-a-time challenges from a standard set, like an obstacle course.
It’s much more like striking out into the wilderness, knowing that your destination is out there in that direction... somewhere... but not exactly how you can get to it or what you’ll encounter along the way.
Trailblazing to the rescue
There are plenty of people who have actually, literally struck out into the wilderness, knowing that their destination is out there in that direction, somewhere, but not exactly how they could get into it or what they’d encounter along the way:
People who are non-metaphorical explorers.
Take, for example, Lewis and Clark1.
They knew the Pacific Ocean was out there to the west. They knew there were rivers, and at least one set of mountains to cross.
To get there, they moved in short increments, gaining a little bit of knowledge at a time. As they progressed, they accumulated a body of knowledge that was infinitely more reliable not only for their own return, but for future travelers, than any detailed-but-speculative map could ever be.
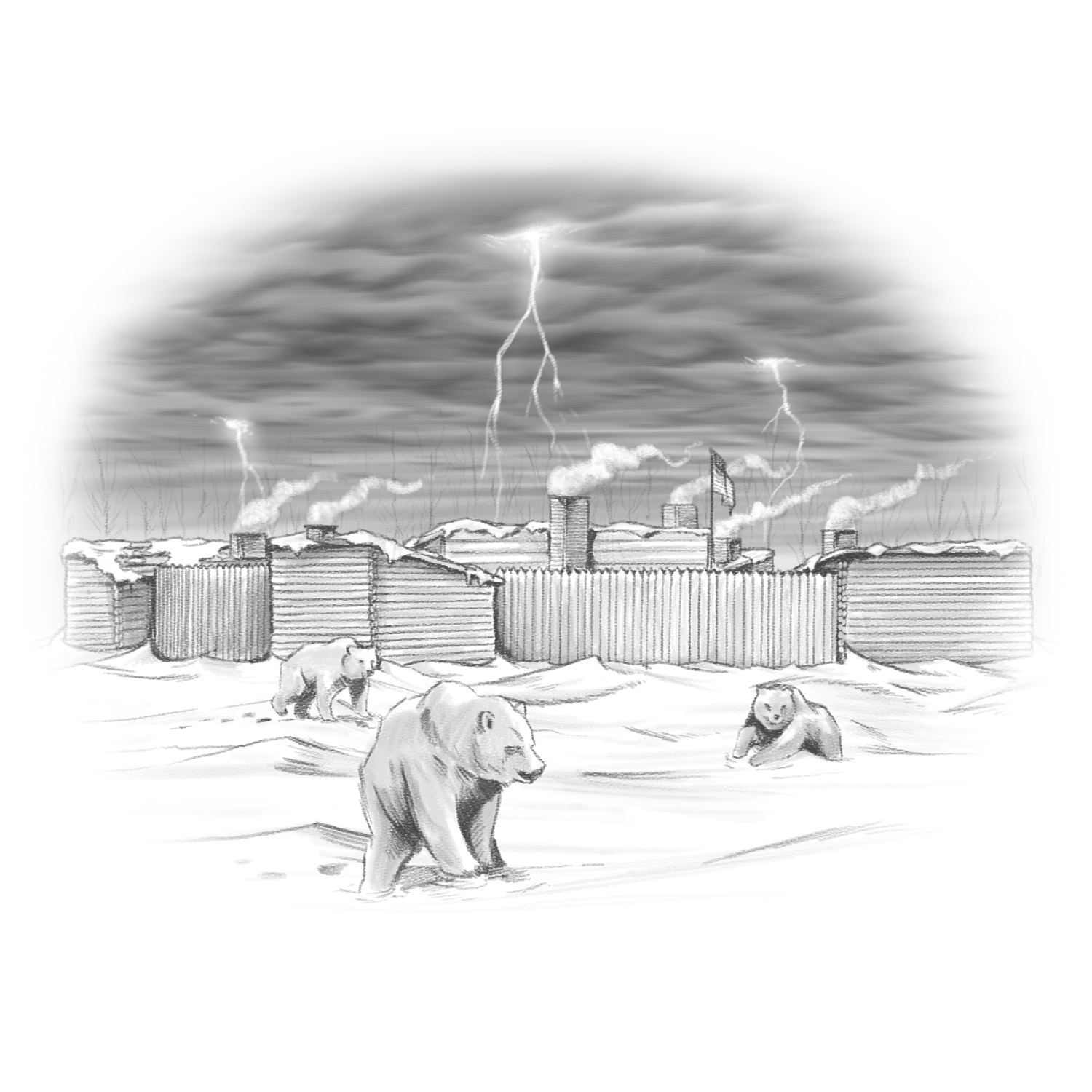
True, Lewis and Clark’s problems aren’t identical to yours. You won’t have to camp in one place all winter, there are no bears in your server room, and no matter how many whitepapers you read about the cloud, a thunderstorm won’t develop in your office.
But thematically their challenges for literal exploring have a lot in common with yours for data quality:
“Yet at the very moment of doing this [Lewis] knew that much of what was offered was based on nothing more than guesswork, dimly understood Indian tales, or academic logic concocted as a substitute for actual observation. On occasion he must have felt completely adrift: how could he stake his success on the reliability of the very charts he was supposed to correct during his travels?”2
It only takes a couple of word substitutions to make this match your data quality situation.
Here’s the main ways in which the trailblazer approach helps you actually achieve your data quality goals:
- Better equips you for encounters with problematic “unknown unknowns.”
- Mitigates the negative effects of intra-company politics on projects.
- Helps you deliver short- and medium-term results, not just long-term.
How to trailblaze data quality
So how do you translate a trek across the continent into a data quality project?
Commit to goals, not to process.
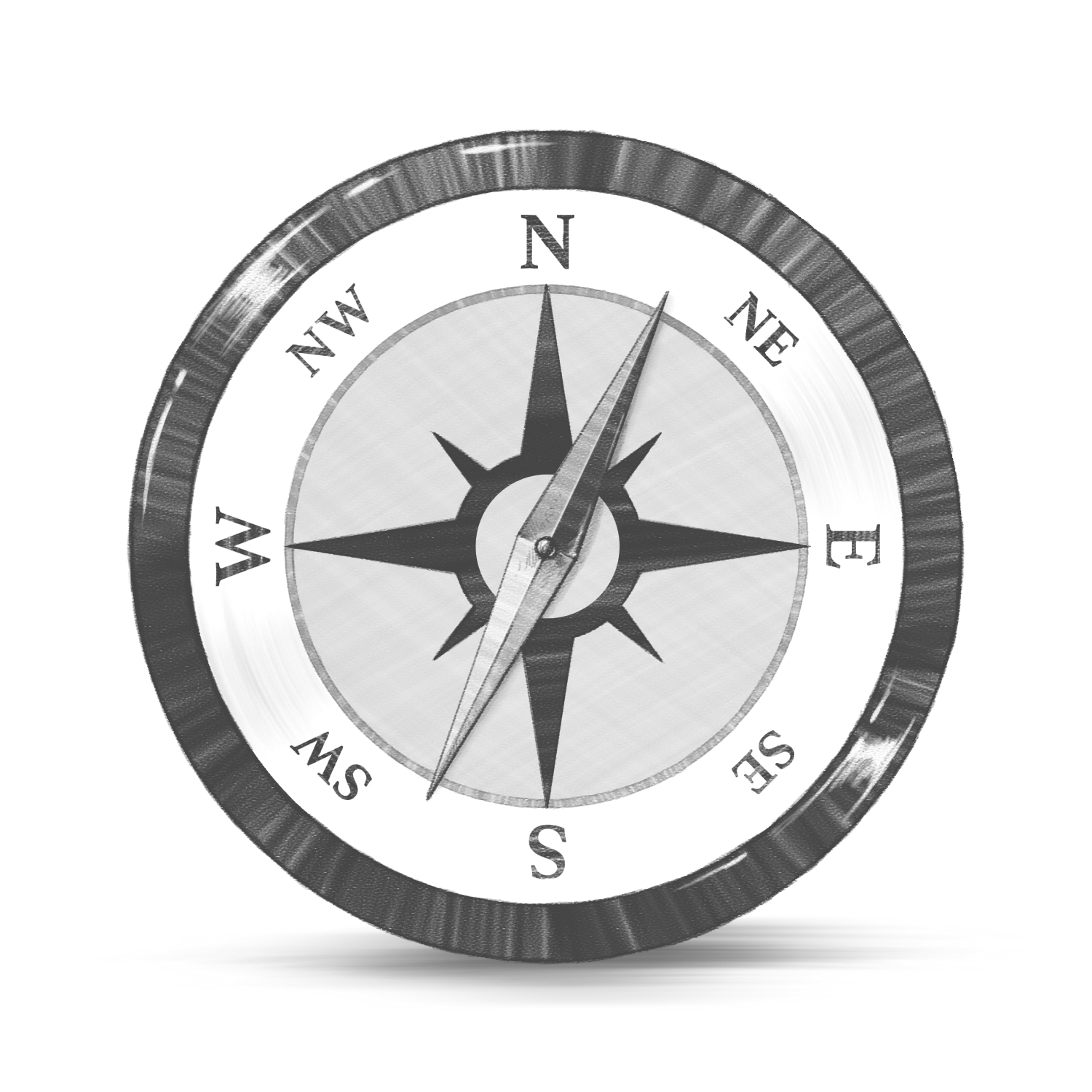
Decide what you want to do, but be flexible about how you do it. Short-term, this means that if the approach you’re using isn’t working out, you should cut your losses and try again.
Long-term, if some aspect of your company changes so that the old process isn’t as effective anymore, this means that you should change up your process. Your data quality should evolve with the company, not hold it back.
Define accomplishments.
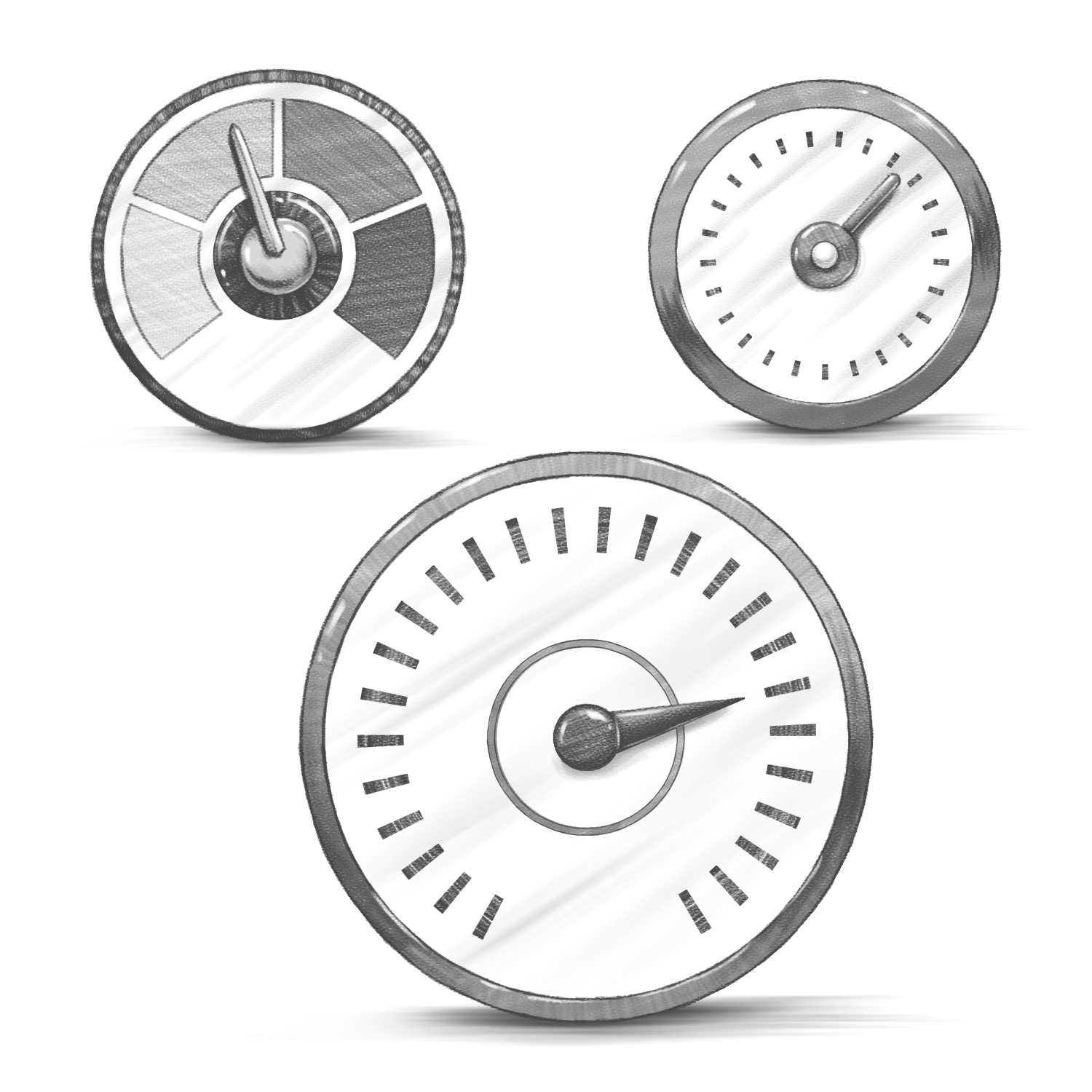
You need concrete, measurable, and above all meaningful goals. That way, you know when you’ve reached it--something that a vague goal like “get data quality” won’t give you.
Accomplishments in data quality should also be grounded in support of business goals, not just completion of arbitrary data-quality-esque tasks.
Be realistic.
We’ve already discussed why a detailed top-down beginning-to-end plan is a bad idea. But that’s not to say we should throw out the whole idea of pre-planning.
Instead, the key is to be realistic in your outset planning and avoid overcommitment.
Interview your day-to-day system and data users to discover what kind of data quality problems they regularly encounter.
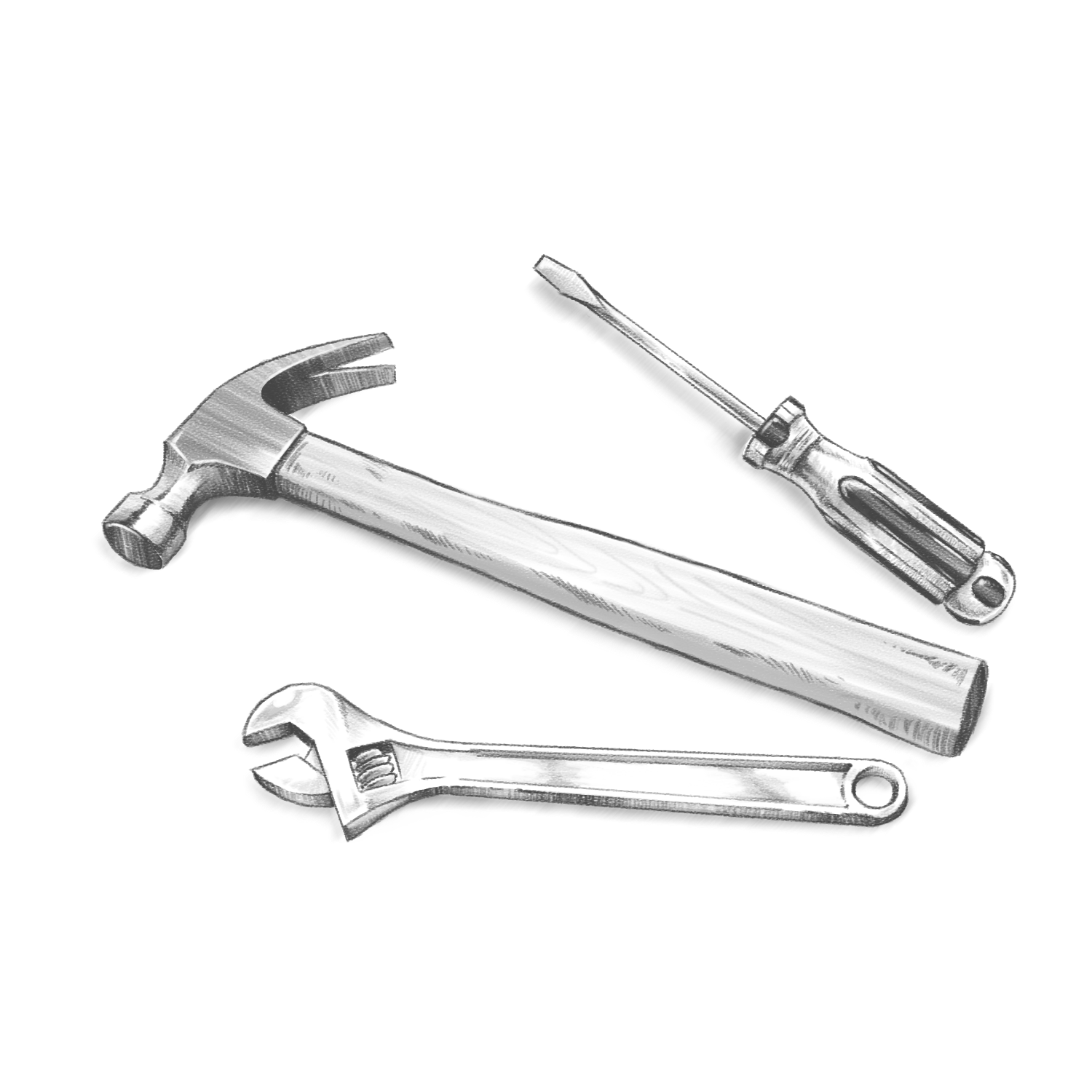
Pick tools that allow you to address as many of those known issues as possible. But make sure that you’ll have other options too--don’t lock yourself into exactly the features you think you need and nothing else.
Choose a beginning goal that’s one of your lower-hanging fruit. Not only will that help you work out your process with relatively low stakes, but its success can help you build support for longer-term projects that can involve more people outside of your team.
Problem-solve.
Data quality is hard in part because the problems and challenges are so different from company to company, and even within the same company over time. You need to embrace a problem-solving mindset that will get you past these hurdles.
Especially for your first few projects, focus on making sure you have the capabilities to perform the fundamentals--finding, exploring, profiling, and extracting data--that are the core tools of data quality.
By keeping these principles in mind as you embark on your data quality journey, you can get faster results, work more effectively, and build a more resilient program.
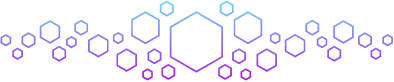
Do you like the trailblazer idea, but don't have the time, people, or know-how to put it into action? Our experts can help: schedule a get-to-know-you with a MIO team member and learn more.
1If you’re unfamiliar: Between 1803 and 1806 Meriwether Lewis and William Clark led an expedition, commissioned by then-president Thomas Jefferson, from St Louis MO to the coast near modern-day Astoria OR, and back.
2David Lavender, The Way to the Western Sea: Lewis and Clark across the Continent, p 29. (emphasis added)